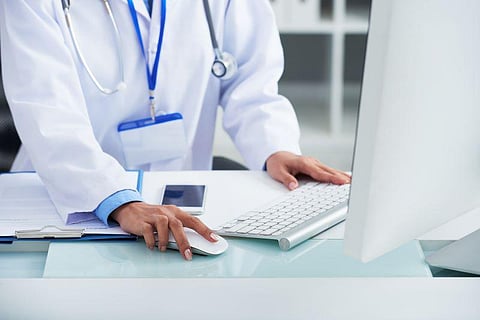
FRIDAY, Jan. 12, 2024 (HealthDay News) -- Large language models (LLMs) can potentially improve the identification of social determinants of health (SDoH) in electronic health records (EHRs), according to a study published online Jan. 11 in npj Digital Medicine.
Noting that SDoH play an important role in patient outcomes but their documentation is often missing or incomplete in EHRs, Marco Guevara, from Mass General Brigham and Harvard Medical School in Boston, and colleagues examined the optimal methods for using LLMs to extract six SDoH categories from narrative text in the EHR: employment, housing, transportation, parental status, relationship, and social support.
The researchers found that the best-performing models were fine-tuned Flan-T5 XL and Flan-T5 XXL for any SDoH mentions and adverse SDoH mentions, respectively. Across models and architecture, there was variation in the addition of LLM-generated synthetic data to training, but this improved the performance of small Flan-T5 models. In the zero- and few-shot setting, the best finetuned models outperformed zero- and few-shot performance of ChatGPT models, except GPT4 with 10-shot prompting for adverse SDoH. When race/ethnicity and gender descriptors were added to the text, finetuned models were less likely than ChatGPT to change their prediction, suggesting less algorithmic bias. Overall, 93.8 percent of patients with adverse SDoH were identified with the models, while International Classification of Diseases-Version 10 codes captured 2.0 percent.
"In the future, these models could improve our understanding of drivers of health disparities by improving real-world evidence and could directly support patient care by flagging patients who may benefit most from proactive resource and social work referral," the authors write.